Digitization of Mining and Mineral Processing Industry
The implementation of digital solutions based on machine learning and computer vision in mining and mineral processing can significantly enhance process efficiency and reduce metal losses. Such high-tech systems have great potential for optimizing and automating various stages of production.
Creating digital twins for mill and flotation process control, determining ore composition, identifying defects and ore contamination, and detecting foam layers are just a few of the possibilities of digitization. One possible solution is the creation of digital twins for mill and flotation process control. A digital twin is a virtual model of a real object or process that allows testing, analysis, and optimization of the system's operation in a virtual environment. Using digital twins improves equipment control and tuning, optimizes processes, and prevents potential metal losses.
The benefits of such digital solutions in mining and mineral processing are evident. They can increase metal production by up to 3% and reduce losses in tailings (in waste material) by up to 20%. The main optimization areas are related to feeding, grinding, and flotation processes, which play a key role in the production cycle.
The implementation of digital solutions in mining and mineral processing requires a comprehensive approach and collaboration between mining experts, production automation specialists, and data analytics professionals.
Ore feeding or ore supply
The implementation of digital solutions for optimizing mining and mineral processing, based on machine learning and computer vision, has several advantages and can significantly enhance process efficiency, greatly improving overall performance.

In the case of feeding mills with ore of varying quality, the use of computer vision systems allows for the swift classification and monitoring of the supplied ore. Cameras and computer vision algorithms can detect ore fragments and determine their composition in real-time. This enables the adjustment of mill operations and control of the feed to ensure stable performance.

The optimization of ore feeding can be complemented by systems for detecting container defects and identifying ore contamination. Such systems are capable of detecting the presence of foreign objects that may damage the mill and providing warnings about potential issues.
Ore Grinding
During the ore grinding stage, mills encounter uneven ore feed and variations in ore hardness, which can lead to overload and emergency shutdowns. To prevent such situations, computer vision and machine learning systems can analyze information from mill cameras, sensors, and conveyors. This allows the construction of mathematical models and the prediction of mill performance, optimizing the ore grinding process. Accurate predictions and optimization help increase productivity and prevent emergencies, contributing to time and resource savings.
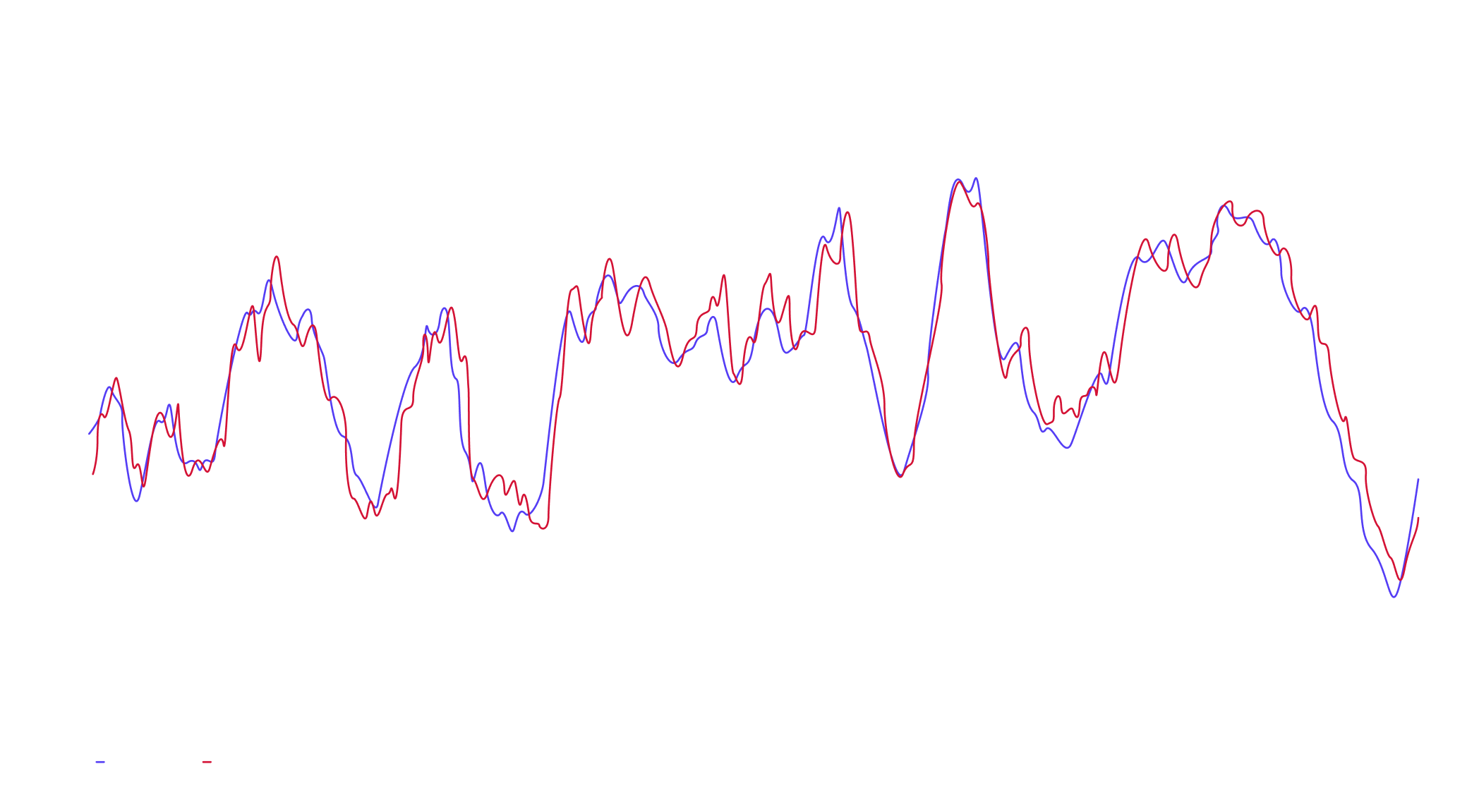
An additional benefit of optimizing mill operations is the increased lifespan of their internal lining, reducing costs associated with replacement and refurbishment.
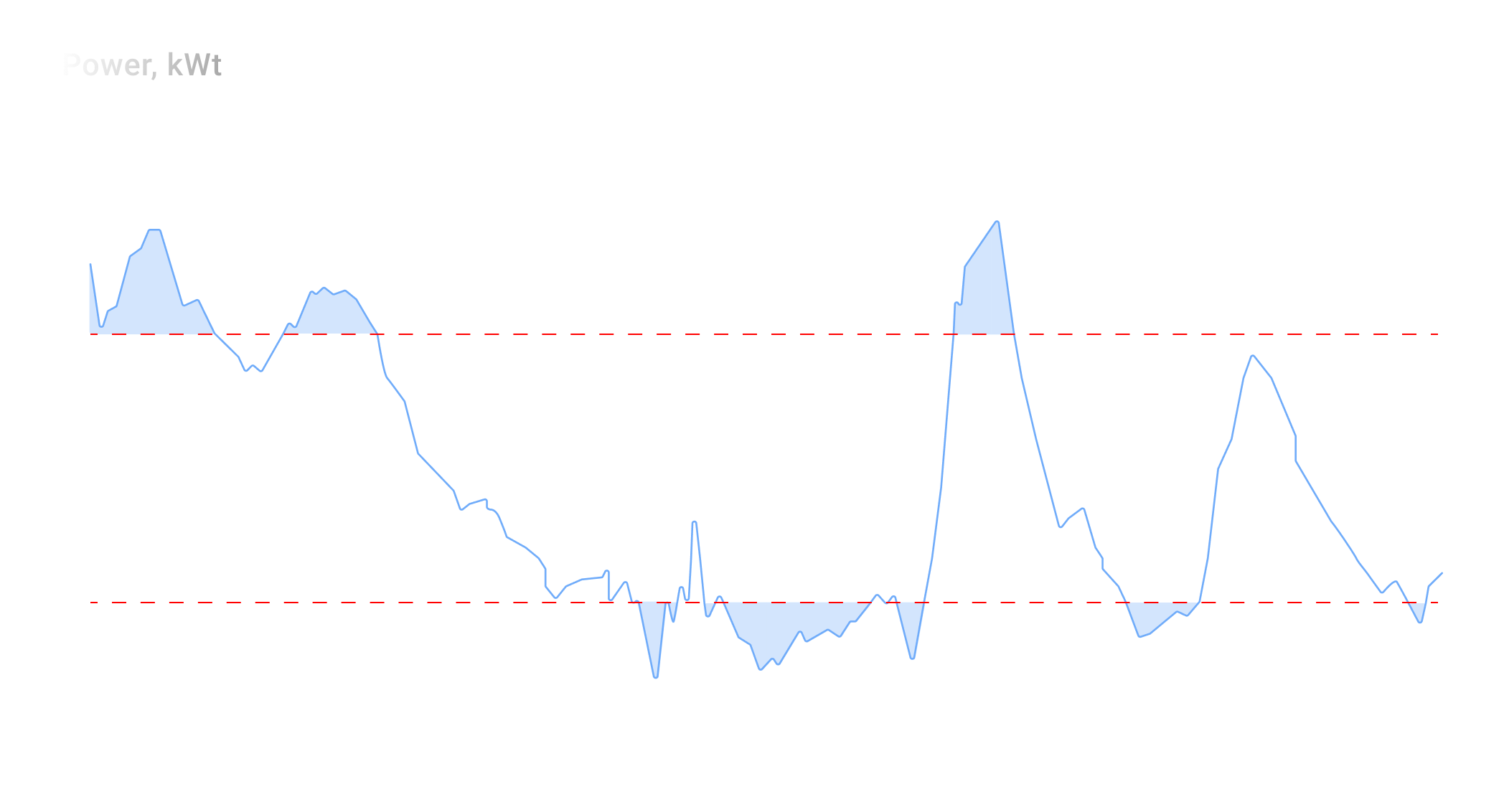
The stable operation of mills also influences the next stage - flotation, ensuring the stability of all processes at the mining and processing plant.
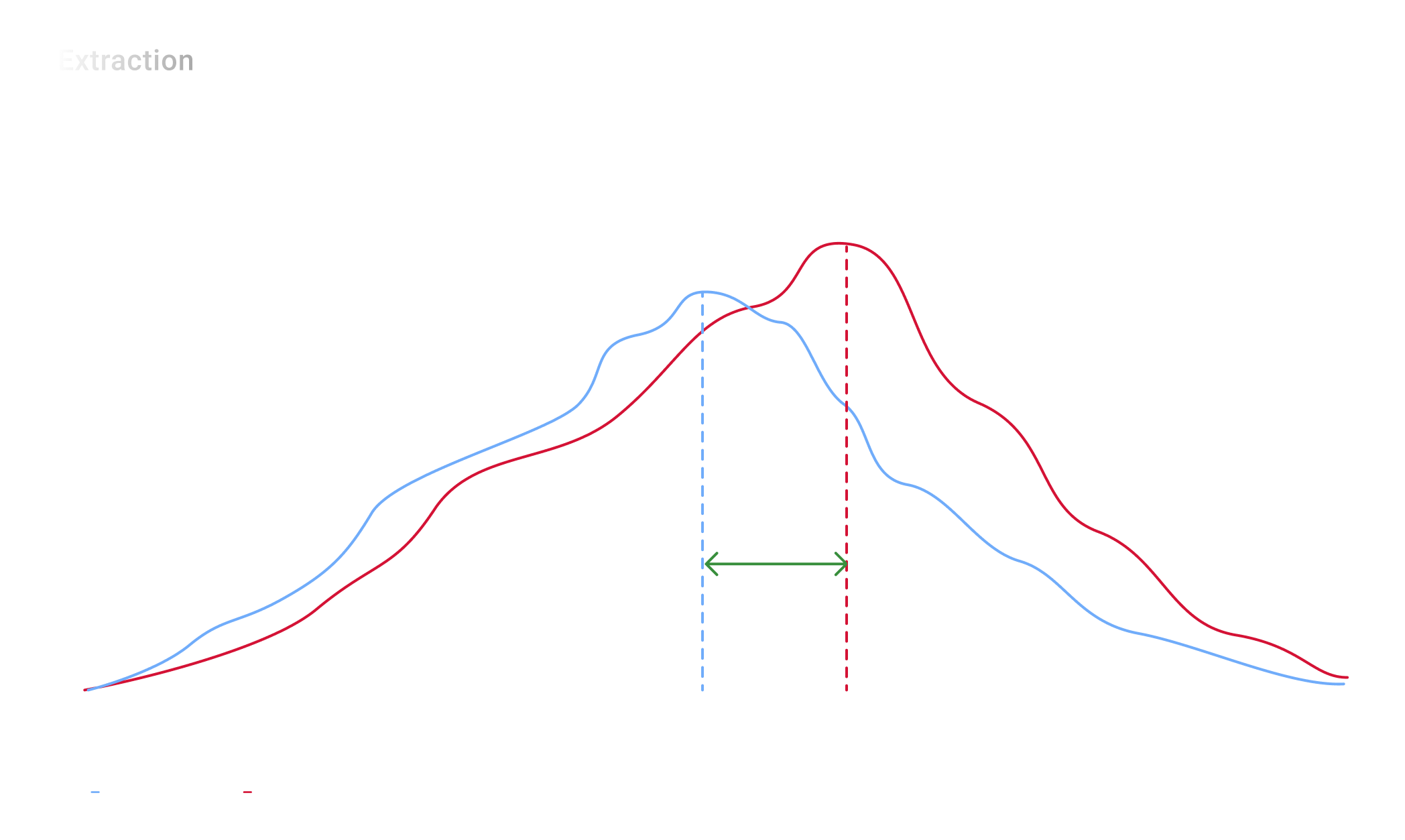
The financial return from such implementation can amount to tens of millions of dollars per year.
Flotation
The goal of the flotation process is to separate minerals into hydrophobic and hydrophilic particles to extract the concentrate and discard the tailings. Hydrophobic particles, which do not readily wet with water, attach to air bubbles introduced into the medium. These particles are then carried into the froth layer at the phase boundary and separated from hydrophilic particles, which wet with water. This way, minerals are separated into valuable components found in the concentrate and less valuable components forming the tailings. It's important to note that there may still be some amount of metals in the tailings, but their concentration will be lower than in the concentrate.
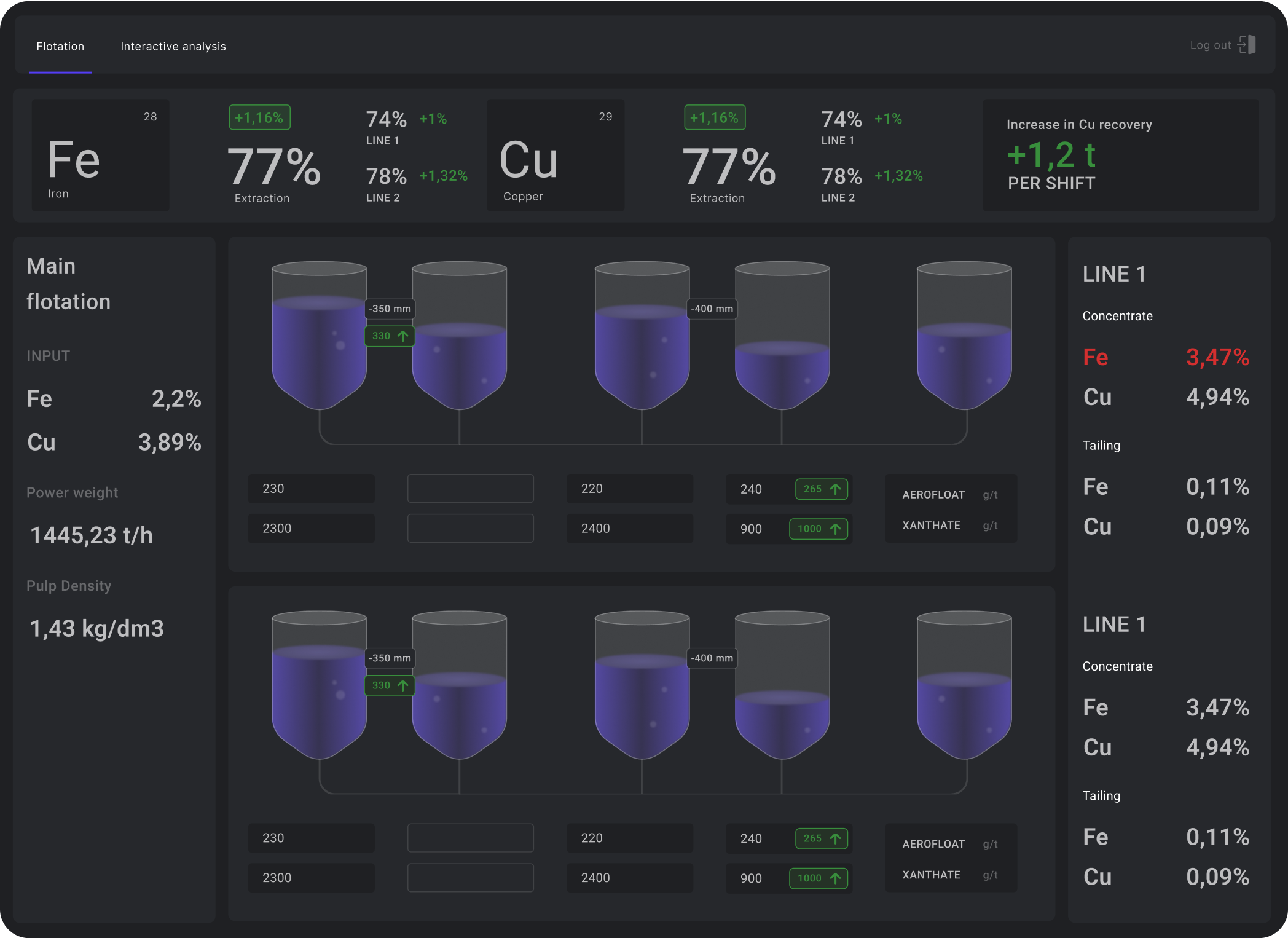
The flotation process can consist of several circuits. One such circuit may include up to 10-20 flotation cells forming a single line, the operation of which depends on numerous parameters:
- Levels
- Air dosing
- Pulp density
- Volumetric material flow
- Metal content in the feed, and more
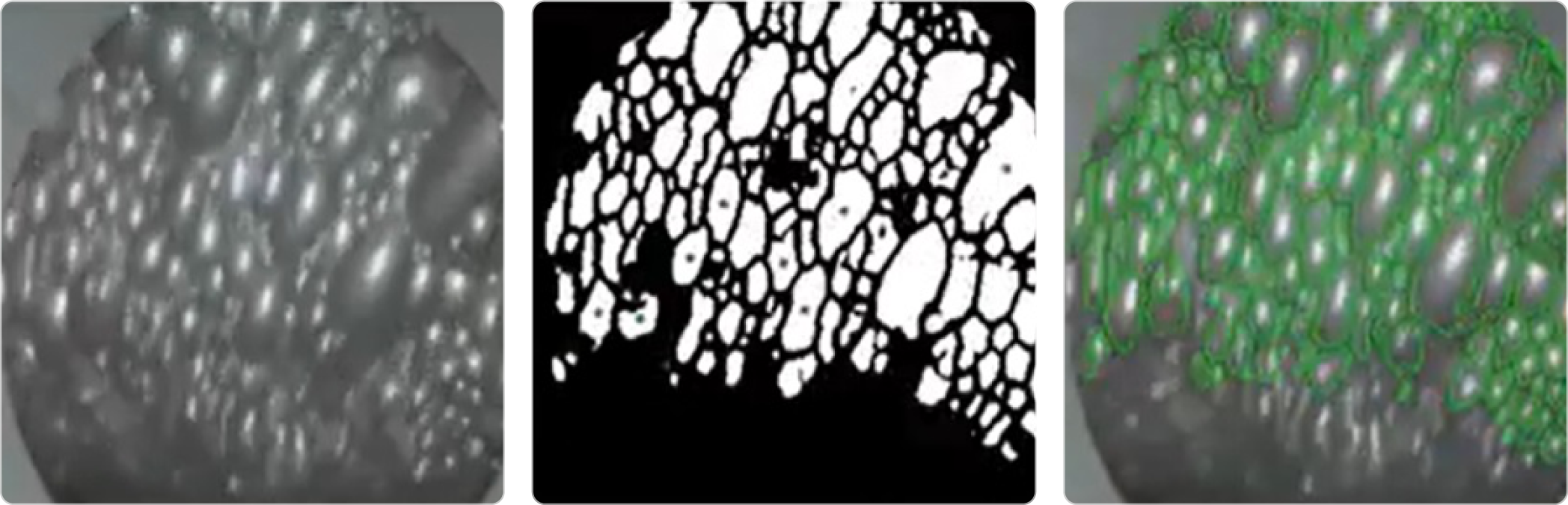
Manually regulating flotation is extremely challenging. Even more challenging is doing it optimally. Inexperience, lack of attention, and different operator tactics can play a role and lead to reduced recovery.
Artificial intelligence (AI) plays a crucial role in optimizing the flotation process and enhancing its efficiency. With AI, mathematical models can be created to monitor the quality of products coming out of flotation machines and make forecasts for several cycles ahead. These models can account for various factors, such as ore composition, process parameters, and operating conditions.
Digital twins can also be used in the context of flotation. They are virtual models or replicas of real systems, including flotation units and their components. Digital twins can serve as guides for operators, providing information and recommendations for process optimization. Moreover, they can be fully integrated into the process automation system (PAS) to enable automatic flotation control based on AI recommendations. This allows sending signals to controllers based on AI recommendations, signaling the need for specific control actions, such as changing reagent dosages, levels, or air, to maximize the recovery of valuable metals.
Thus, AI and digital twins provide the opportunity for the automation and optimization of the flotation process, increasing efficiency and improving control over production parameters.
The implementation of a flotation optimization system in mining and mineral processing can lead to a range of significant results and advantages:
- Increased extraction of valuable metals: Through the use of artificial intelligence and digital models, the optimization system allows maximizing the extraction of precious metals. This is achieved through precise control and optimization of flotation process parameters, such as levels, air dosing, reagents, and others.
- Maintaining metal content in the concentrate within specified ranges: The optimization system provides precise control of the flotation process, allowing for the regulation of metal content in the concentrate within defined limits. This is crucial to ensure the quality and compliance with the requirements of the final products.
- Minimization of metal content in tailings: Optimizing the flotation process allows for reducing the metal content in tailings, which is economically efficient and helps minimize the loss of valuable resources.
- Operator guidance: Digital models and the optimization system can be used as guides for operators, providing recommendations and suggested control actions. This helps enhance the operators' skills and ensures the stability and optimality of the flotation process.
- Financial return: Implementing a flotation optimization system can lead to significant financial gains. On average, increasing the extraction of precious metals by 0.3-2% can result in an additional annual revenue of $10-30 million.
- Froth layer detection: An optional feature in the flotation stage is the detection of the froth layer. This allows for real-time monitoring of froth parameters, optimizing the flotation operation, and preventing unexpected situations.